The Essential Guide to Machine Learning Data Labeling
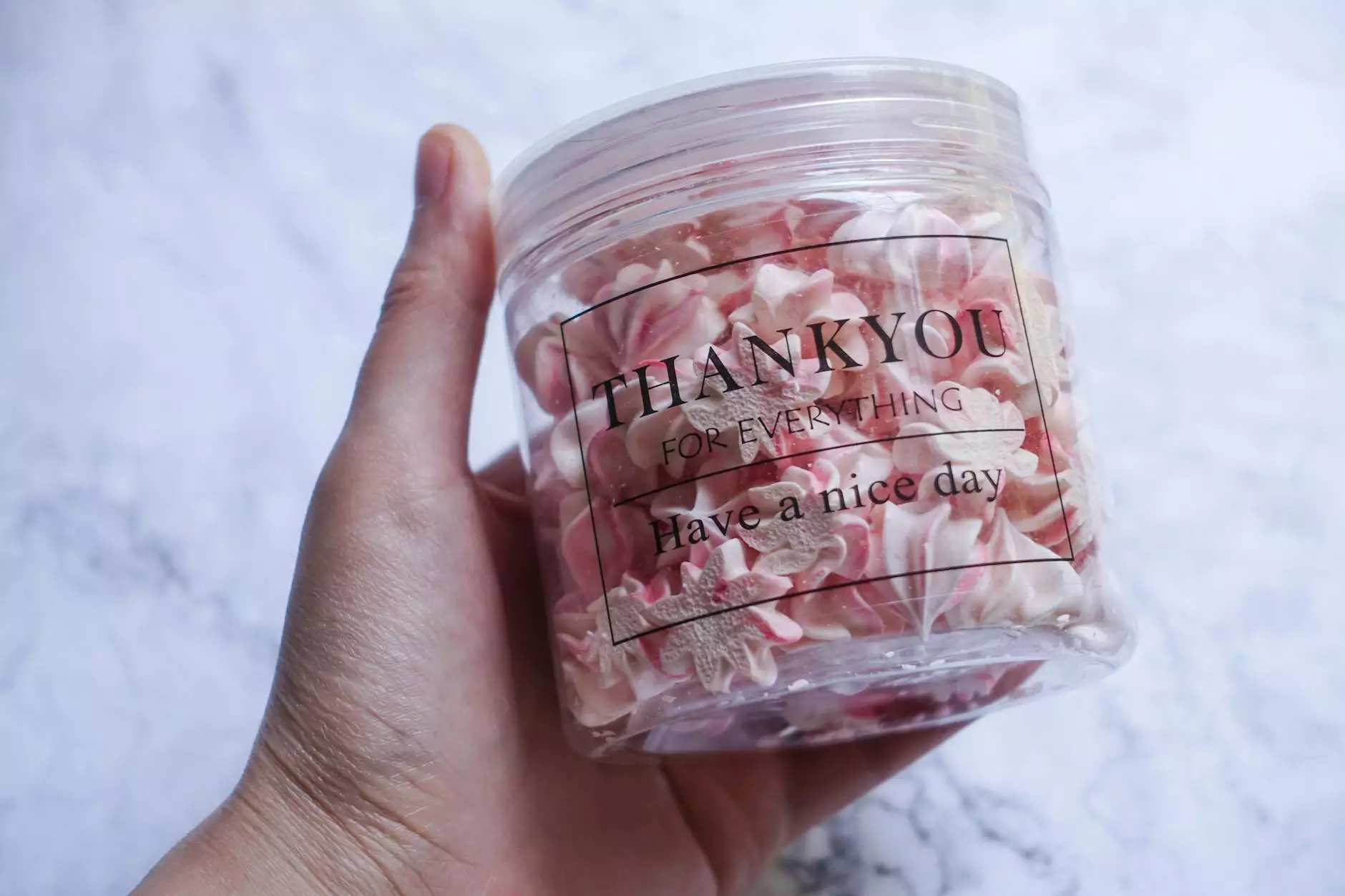
In the rapidly advancing world of artificial intelligence (AI) and machine learning, data labeling has become an indispensable process that drives the success of various applications. Businesses relying on cutting-edge technologies are keenly aware that the quality of their machine learning models is only as good as the data fed into them. Therefore, mastering the intricacies of machine learning data labeling is critical for any organization looking to harness AI effectively.
Understanding Machine Learning Data Labeling
At its core, machine learning data labeling refers to the process of annotating or tagging data so that machine learning algorithms can understand it. This process is essential for supervised learning, where models learn from labeled data to make predictions or classifications.
Why is Data Labeling Important?
The significance of data labeling cannot be overstated. Here are some compelling reasons:
- Model Accuracy: Clearly labeled data enhances the accuracy of AI models, allowing them to perform better in various tasks.
- Training Time: Properly labeled data reduces the time taken to train machine learning models, leading to faster deployment.
- Scalability: As businesses grow, the volume of data increases. Well-organized labeled datasets can scale with the organization's needs.
Types of Data for Labeling
Data labeling can be applied across various types of data. Here are the most prevalent categories:
1. Image Data
Image labeling involves tagging images with relevant labels or bounding boxes. This is crucial for tasks like object detection, facial recognition, and autonomous vehicles.
2. Text Data
Text labeling encompasses tagging words, phrases, or entire documents. This is especially beneficial for Natural Language Processing (NLP) tasks, sentiment analysis, and chatbots.
3. Audio Data
Audio labeling requires transcripts or tagging parts of audio for speaker recognition, language detection, and voice command understanding.
4. Video Data
Video data labeling involves marking specific frames within a video, which is vital for surveillance, action recognition, and video segmentation tasks.
Best Practices for Machine Learning Data Labeling
When engaging in data labeling for machine learning, following best practices ensures that the results are accurate and useful.
1. Define Clear Guidelines
Establish comprehensive labeling guidelines that delineate the process, providing labelers with a clear understanding of what is expected.
2. Use Quality Control Measures
Incorporate quality checks throughout the labeling process to identify and correct inaccuracies. This can be achieved through regular audits, double-checking, and employing multiple labelers for the same data.
3. Choose the Right Tools
Utilize sophisticated data labeling tools that can streamline the process, reduce time, and enhance accuracy. Tools like Keymakr offer platforms tailored for effective data annotation.
4. Leverage Automation
While human input is invaluable for nuanced labeling, utilizing automation for preliminary labeling can significantly speed up the process. Machine learning algorithms can assist with initial predictions, which humans can then refine.
The Role of Technology in Data Labeling
As technology progresses, so do the methods employed in data labeling. Here are some technological advancements that are shaping current practices:
1. Artificial Intelligence
AI aids in automating repetitive tasks within data labeling, reducing the burden on human annotators and speeding up the process.
2. Crowdsourcing
Many companies are leveraging crowdsourcing platforms to gather large-scale data labeling efforts from skilled individuals across the globe, increasing both volume and diversity.
3. Cloud Computing
Cloud solutions allow for scalable, collaborative data labeling projects, enabling teams to work together efficiently regardless of their geographical location.
Common Challenges in Machine Learning Data Labeling
Data labeling is not without its challenges. Below are some common obstacles organizations face:
1. Volume of Data
The sheer amount of data available can be overwhelming and difficult to manage. Organizations need efficient systems in place to handle this vast quantity of information.
2. Complexity of Tasks
Some tasks may require specialized knowledge or expertise, making it challenging to find the right talent for accurate labeling.
3. Quality Assurance
Ensuring consistent quality across labeled data is vital. Without it, machine learning models may produce unreliable results.
Conclusion: The Future of Machine Learning Data Labeling
The field of machine learning data labeling is evolving, and its importance cannot be emphasized enough. As AI technologies continue to grow and diversify, the demand for well-labeled datasets will also increase. By following best practices, leveraging technology, and remaining vigilant about quality, businesses can ensure they stay ahead of the curve.
Investing in effective data labeling strategies will not only enhance the performance of machine learning models but also position organizations as leaders in the field of AI innovation. With platforms like Keymakr, companies can streamline their data labeling processes, ensuring high-quality outputs that drive intelligent business solutions.
For more information on how to enhance your machine learning projects with effective data labeling strategies, visit Keymakr.