Unlocking the Future of AI with a Data Labeling Tool
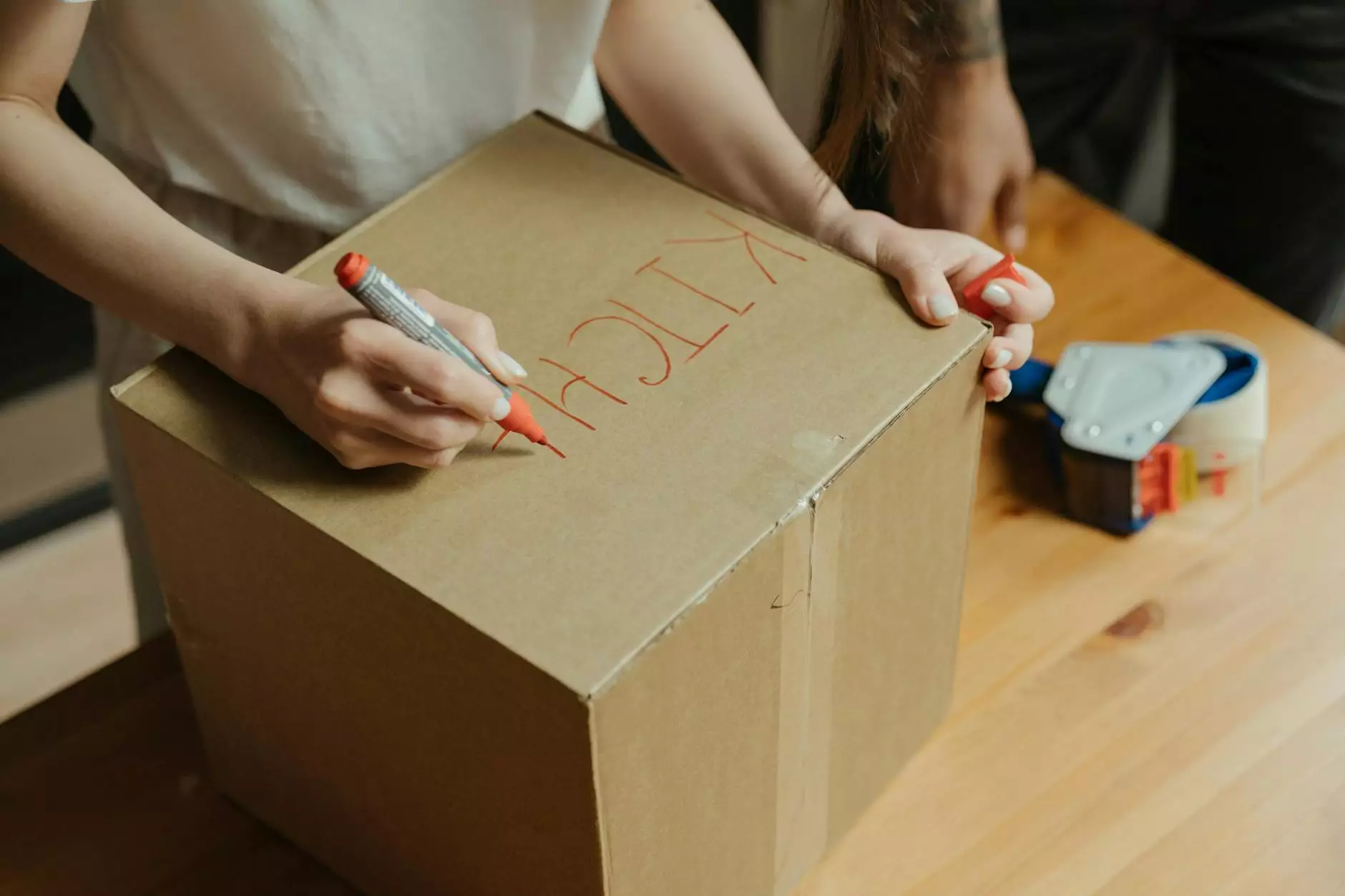
In the rapidly evolving sector of artificial intelligence (AI), the necessity for precise and efficient data management cannot be overstated. One of the critical components to achieving high performance in AI projects is the utilization of a data labeling tool. This article delves into the significance of data labeling tools, their functionalities, and how they create a competitive edge in the field of software development.
What is a Data Labeling Tool?
A data labeling tool is a software application designed to tag or annotate data—such as images, text, audio, and video—so that machine learning models can learn from it. Accurate labeling is fundamental as it allows AI systems to make informed decisions based on the tagged information. Various tools are available, each optimized for different types of data and use cases.
Key Features of Data Labeling Tools
- User-Friendly Interface: Modern data labeling tools often feature intuitive interfaces that facilitate ease of use.
- Automation Capabilities: Advanced tools incorporate machine learning algorithms to assist with preliminary labeling, significantly reducing the time and effort required.
- Collaboration Tools: Many tools allow teams to work collaboratively, making it easy to review, edit, and finalize data labels.
- Quality Assurance Mechanisms: Reliable tools include features for validating and monitoring the accuracy of data labels.
Importance of Data Labeling in AI
The importance of data labeling in artificial intelligence and machine learning can be outlined through several key points:
- Data Quality: High-quality labeled data is essential for training models that perform well in real-world applications.
- Model Accuracy: The performance of any AI model hinges on the quality of its training data; incorrect labels lead to poor predictions and inaccurate outcomes.
- Scalability: Efficient labeling processes powered by the right tools can help companies scale their AI projects effectively.
Applications of Data Labeling Tools
Data labeling tools find application across various domains, including:
1. Image Recognition
In the world of computer vision, data labeling is critical for tasks like object detection, image segmentation, and facial recognition. Labels might include bounding boxes around objects or segmentation maps that delineate different areas of an image.
2. Natural Language Processing (NLP)
For NLP tasks, data labeling can involve tagging parts of speech, sentiment analysis, or entity recognition. This helps in developing chatbots, language translation services, and sentiment analysis tools.
3. Autonomous Vehicles
In the automotive industry, labeled data is crucial for training models to detect pedestrians, traffic signs, and lane boundaries. This is a matter not just of efficiency, but also of safety.
4. Healthcare
In healthcare, annotated data is used to improve diagnostics and personalized medicine. Bioinformatics relies heavily on accurate data labeling for genetic and protein analysis.
Choosing the Right Data Labeling Tool
When selecting a data labeling tool, consider the following factors:
- Type of Data: Choose a tool that specializes in the type of data you need to label—be it images, text, or audio.
- Customization Options: A good tool allows you to customize labels according to your project’s requirements.
- Integration: Ensure the tool can integrate smoothly with your existing workflows and technology stack.
- Cost: Consider your budget and the pricing models of the tools. Some tools offer pay-as-you-go options, which can be beneficial for smaller projects.
Benefits of Using a Data Labeling Tool
Implementing a reliable data labeling tool can yield numerous benefits:
1. Increased Efficiency
Automating the labeling process through advanced tools reduces the time taken to annotate data. This means faster project completion and quicker time to market.
2. Enhanced Collaboration
Many tools support multiple users, enabling teams to collaborate effectively. This collaborative approach enhances the quality and consistency of labeled data.
3. Improved Outcomes
With access to quality assurance features, teams can ensure that data labels are accurate, leading to better-performing AI models.
4. Cost-Effectiveness
While there may be upfront costs associated with these tools, the long-term savings in manual labor and faster project completion can offer a high return on investment.
Top Data Labeling Tools in the Industry
The market is replete with data labeling solutions. Below are some of the top contenders that stand out for their features and adaptability:
1. Keymakr
Keymakr is a powerful data labeling tool known for its user-friendly interface and comprehensive support for various data types including images, text, and audio. Its automated labeling capabilities and collaboration features make it a top choice for teams looking to streamline their data preparation process.
2. Labelbox
Labelbox offers a collaborative data labeling platform equipped with tools for workflow management. It provides customizable labeling interfaces, which can be tailored to specific project needs.
3. Amazon SageMaker Ground Truth
This tool from Amazon Web Services helps in building highly accurate training datasets for machine learning. It provides workflows that facilitate the easy creation of labeled data through a combination of manual and automated processes.
4. Supervisely
Supervised learning is simplified with Supervisely, which supports image and video annotation, as well as 3D point cloud labeling. Its powerful community features also offer users access to shared datasets.
Conclusion
In conclusion, a powerful data labeling tool is indispensable in the software development landscape, especially within AI projects. The accuracy, efficiency, and quality of data labeling are paramount for the success of machine learning models. As AI applications continue to proliferate across various industries, leveraging the right tools will determine the effectiveness and reliability of the resulting systems. Investing in a robust data labeling solution is not just a strategic decision; it's a necessary step towards unlocking the future of intelligent applications.